The Data Revolution: How Real-World Evidence is Changing Pharma
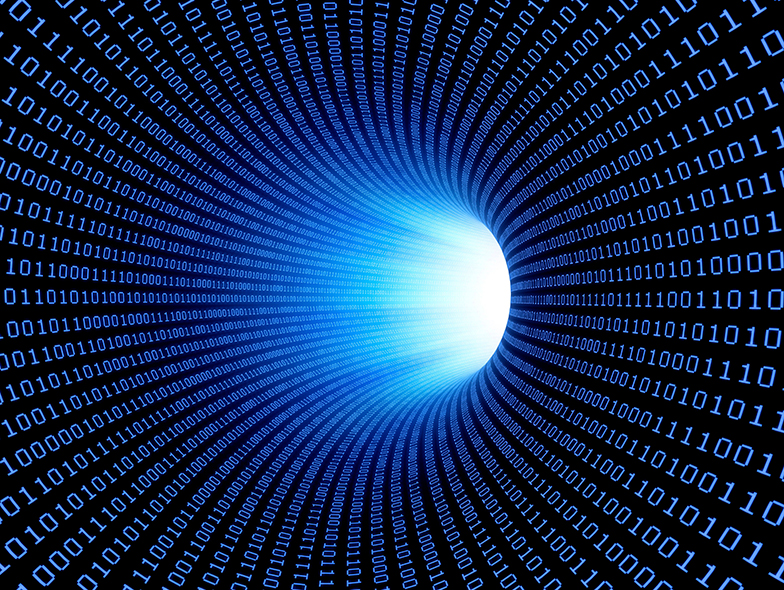
A look at the companies extracting valuable insights from health data to accelerate clinical development and support regulatory decision making.
In the life sciences industry, real-world data is increasingly being used to improve clinical trial designs, support regulatory decision making and monitor drug safety post-approval, among other applications.
The rise in smart devices, such as wearables and other biosensors, means there is more health-related data available than ever before, and with it a myriad of opportunities for drugmakers and regulatory agencies willing to leverage its potential.
In this article, we will explore the innovative ways data is being used to transform several aspects of drug development, as well as considering the challenges posed by this new frontier.
How did we get here?
Interest in the use of real-world data (RWD) and real-world evidence (RWE) to support drug development first emerged in the United States when Congress passed the 21st Century Cures Act of 2016[i]. The goal of the Act was to accelerate drug development and supplement traditional clinical trial data with data from a more diverse array of patients in a wider range of settings[ii]. Under the Act, the US Food and Drug Administration (FDA) was instructed to publish a framework for evaluating the use of RWE in regulatory decision making, which it did in December 2018. It has since published several pieces of draft guidance on the subject.
While interest in RWD and RWE has been growing for several years, it was during the COVID-19 pandemic that decision makers pivoted from reluctance to willingness to rely on RWE for policy and regulatory decision making. Without time for blinded, controlled clinical trials, the FDA found itself considering data sources that it would never previously have relied on for such decisions. And while some of those choices have been criticised in hindsight, this approach made the rapid development of vaccines and therapeutics possible[iii].
Where does the FDA stand?
The challenge for the FDA is ensuring that the RWD used to generate RWE is high quality, relatively complete and — for post-approval studies in particular — is suitable for emulating the data obtained in actual clinical trials[iv]. After publishing its Framework for Real World Evidence Program in 2018, the FDA followed up with seven pieces of guidance and two publications on the subject[v]. Of the seven guidance documents, four were published in the final quarter of 2021.
Though non-exhaustive, these documents provide a solid jumping-off point for innovators hoping to use RWE to support their regulatory submissions. In her analysis of the recent guidance, Quanticate’s Karen Ooms said the FDA’s overall aim was to clarify expectations around data usage, as well as highlighting the importance of data quality and integrity. Ms. Ooms is Executive Vice President, Head of Statistics at the data focused CRO.
Speaking to CPHI, she explains: ‘The requirements for RWD must be relevant and reliable. To be relevant there should be sufficient detail, data can be analysed using sound statistical techniques and can be interpreted using scientific judgement. This relevant data can include patient characters, exposures and outcomes.
‘In terms of reliability, we are talking about how the data was collected. We look at whether the processes in place during data collection and analysis are robust so that errors are minimized, and that data quality and integrity is sufficient.’
Ooms also notes that there is a need for individual source data verification and some sources, such as wearable devices and eCOA patient diaries, require ‘careful thought’.
At the beginning of 2022, the FDA said it intends to release further guidance on designing studies that use RWD, specifically externally controlled trials and randomised controlled trials conducted in clinical practice settings. It added that while the agency is already using RWE for regulatory decisions, it would ‘consider how to optimally incorporate RWD and RWE to provide trustworthy information about the safety and effectiveness of drug therapies’ going forward[vi].
Current applications - How is the data being used?
RWD and RWE have many potential applications in clinical development, regulatory decision making and post-approval studies. In this section, we hear from some of the companies leading the charge in this space.
Case Study #1 – Trial design, site selection and patient recruitment
Company: Verana Health
Historically, actions needed to initiate a clinical trial – like patient enrolment, physician engagement and site selection – have been relatively inefficient, requiring several disparate manual processes. But increasingly drug sponsors are utilising RWD to build stronger, more diverse patient cohorts and rapidly identify the most suitable trial sites. To do this, many sponsors turn to companies who curate data from across a range of sources and provide them with actionable insights.
One such company is Verana Health, who partners with medical societies to collect and curate data relating to more than 20,000 healthcare providers from 70 electronic health record (EHR) systems to generate quality real-world data, which the company calls ‘Qdata’. This data can then be leveraged to identify patient populations for clinical trials, as well as the physicians and sites that best correspond with these populations.
Verana Health’s CEO, Sujay Jadhav, explains: ‘When you look at study design, you usually use inclusion/exclusion criteria to identify the type of patients who fit enrolment criteria. Because we have our VeraQ database of about 90 million de-identified patients, you can apply inclusion/exclusion criteria at scale to interrogate the database.
‘In essence, we help to simulate the potential patient population that you’re looking to recruit up front by using artificial intelligence techniques. As you can imagine, that can reduce a lot of downstream inefficiencies.’
Using this data, the company then assists sponsors with site selection and patient recruitment.
‘What we do then is highlight patients who are a good fit for a particular clinical trial to the physician, using our secure Verana Trial Connect software. So, we make it very easy for the physician to recruit patients,’ Jadhav says.
‘Then further along when you are looking to launch a particular drug, we can help identify geographies with patient populations that may benefit from the approved therapeutic, which could help improve patient outcomes.’
Verana Health focuses on the three core therapeutic areas: ophthalmology, urology, and neurology.
Case Study #2 – Enhancing patient insights
Company: Linguamatics, an IQVIA company
Linguamatics, an IQVIA company, leverages its Natural Language Processing (NLP)-based AI platform for a range of applications in the life sciences industry, including drug discovery, clinical research and regulatory compliance. The company’s central focus is on taking unstructured text and extracting useful information from it.
‘It means anywhere you have unstructured text, instead of having to manually read and find the facts you're interested in, you can float those to the surface,’ explains Jane Reed, Director, Life Sciences, IQVIA.
It is estimated that 80% of available data is unstructured, or free text. In order to use conventional tools, methodologies and skills to analyse this data, information buried in the unstructured text needs to be uncovered and converted to a structured format.
Linguamatics’ recent work with Roche serves as just one example of how unstructured text can unlock useful insights. While developing a drug to treat Parkinson’s Disease, Roche sought to broaden its understanding of the conceptual disease model and more accurately capture the voice of the patient. To do this, Roche’s researchers established a series of NLP-based text mining queries to analyse patient discussions around Parkinson’s across several social platforms, blogs, patient forums and relevant websites. As a result of this approach, Roche uncovered several additional symptoms and added two new impacts to the conceptual disease model[vii].
Speaking about Roche’s results, Jane Reed says: ‘The good thing about patient surveys is that they're very rich, they're very deep, but you can maybe only talk to 20 or 50 people because of the process of getting them set up. Roche wanted to know - how can we get a different view of the patient to feed into that clinical disease model?
‘Social media can bring new information into the traditional model, which generally includes a literature search and patient survey. It allows us to get that full landscape of information around how best to design clinical trials, how best to measure outcomes, how to ensure those outcomes are relevant to patients as opposed to the current clinical understanding. Because there are things that patients might not be reporting in a structured medical interview, but they'll be chatting about on social media.’
Case Study #3 – Mapping the patient journey
Company: Arcadia
Healthcare data is fragmented by nature – spread across electronic health records, claims records, and disease registries, among other data silos. Unsurprisingly, this data is far more powerful when it can be brought together to give a holistic overview of a patient’s journey. However, non-standardisation of data and lack of interoperability between systems makes this hard to do.
As a result, many companies are partnering with data specialists who can combine and curate this data for them. One such company is Arcadia.io, a US-based firm that works with healthcare providers and researchers to de-duplicate patient identities across global systems and bring everything together into a clinically relevant dataset - giving a ‘360-degree view’ of the patient.
According to Jim Robbins, Senior Vice President, Life Sciences at Arcadia, unifying patient data is an important aspect of meeting data quality standards set by the FDA.
‘We can follow patients across providers and sites. That's an important dimension because it increases - in the FDA's terms - the longitudinality of the real-world data, because we have more of the patient journey. Longitudinality is a key dimension,’ he says.
The idea of longitudinality intersects with two important concepts mentioned in the FDA’s recent guidance: missingness (how complete is the data?) and provenance (where did the data come from?). In order to ensure the curated data is of sufficient quality, it is important for companies like Arcadia to understand the systems their providers are working with, as well as variables that impact initial data capture.
‘Knowing how a system is customised for a provider and the nature of the data collection for each of those clients allows us to better understand the missingness, completeness and provenance. So, we work with our provider colleagues to know more about those sources. The more we know, the more we can render judgement. And sometimes that means not including data in the research product because it doesn't meet certain completeness standards,’ Robbins explains.
And although much progress has been made to create a ‘complete’ picture of a patient’s health journey, challenges remain in this highly fragmented landscape.
‘It is real world data, and even though we may capture a larger percentage of the patient journey, we still don't know what we don't know. We don't know when the patient may have gone out of network. For example, many patients are reticent to go to a known provider and even to process a claim through their employer for a mental health condition, or for certain lifestyle drugs. They will go off grid because they are reluctant to have any mention of that in their medical record,’ Robbins explains.
‘So, there's leakage that occurs for various reasons. It's not because the EMR isn't doing its job, or the provider isn’t doing its job. It's really because of the patient's decisions.’
Looking forward – What does the future hold for RWE?
In 2018, former FDA Commissioner Scott Gottlieb said RWE had ‘the potential to make our new drug development process more efficient, improve safety and help lower the cost of product development’[viii]. Since then, several companies have made strides to realise that potential.
Bristol Myers Squibb has used RWE to support US and EU marketing applications for three of its products - Opdivo, Abecma, and Orencia[ix] - while the FDA approved a new indication for Astellas Pharma’s Prograf on the basis of a ‘non-interventional study relying on fit-for-purpose RWD’ in 2021[x].
Similarly, Japan’s Pharmaceuticals and Medical Devices Agency approved the use of Pfizer’s lorazepam based on a RWD study among patients outside the country, after a clinical trial in Japan could only enrol one patient. The use of RWD in regulatory decision-making is also a strategic goal for the European Medicines Agency, though these efforts are impeded by the EU’s data privacy regulations and the lack of a standard data platform such as the FDA Sentinel Initiative[xi].
For companies hoping to leverage RWE for their own purposes, the first step is to identify a specific research question or evidence gap that the use of real-world data is trying to address. For example, the use of an RWE study may be most appropriate where randomized controlled trials are not feasible or ethical[ix].
Once that research question has been identified, the FDA recommends engaging the relevant regulator before conducting the study and ensuring you are in alignment on data quality standards and best practices. The FDA’s RWE framework and guidance documents also provide a solid foundation for this, but it is worth remembering that these are general, non-exhaustive recommendations.
Speaking about the guidance, Verana’s Sujay Jadhav notes: ‘The way I look at it, this is the first step in creating a framework in the real-world data evidence arena. I think it's definitely marching towards best practices, and I think it’s going to continue to be a journey, given that this space continues to evolve in a rapid fashion.’
Only time will tell how exactly that journey will unfold, but at this juncture the future looks bright for RWE in pharma.
References
[I] https://www.congress.gov/bill/114th-congress/house-bill/34
[v] https://www.fda.gov/science-research/science-and-research-special-topics/real-world-evidence
[x] https://www.reutersevents.com/pharma/access-and-evidence/future-bright-rwe-pharma-approvals
[xi] https://www.reutersevents.com/pharma/access-and-evidence/future-bright-rwe-pharma-approvals
Related News
-
News mRNA therapy for ovarian cancer and muscle wasting
Researchers demonstrate results of a promising mRNA therapy for ovarian cancer and muscle wasting caused by cachexia, a condition associated with various types of cancers and chronic diseases. -
News Pfizer CentreOne Content Refinement Q3 media buy
For 40 years Pfizer CentreOne has been guiding drug projects to success. Here’s how our services make us an altogether different kind of CDMO: -
News Gut instinct: molecular link between COVID-19 and serotonin cells in the gut
New research may provide further evidence of the gut’s role in SARS-CoV-2 infection and disease severity with a molecular link between serotonin-producing cells in the gut and COVID-19 disease severity. -
News First treatment for Acid Sphingomyelinase Deficiency approved by US FDA
Enzyme replacement therapeutic Xenpozyme has received US FDA approval with an orphan drug designation to treat symptoms of Acid Sphingomyelinase Deficiency, a rare genetic disease. -
News Moderna sues Pfizer/BioNTech over COVID-19 vaccine patent infringement
The pharmaceutical companies behind the leading COVID-19 vaccines are in a legal dispute over intellectual property rights surrounding the mRNA vaccines that were released after emergency use authorization in December 2020. -
News Accepting novel excipients for innovative drug development
With the rapid development and manufacturing of new drugs and therapeutics being pushed through the pipeline, excipient manufacturers are navigating a challenging landscape to meet the demand for new and novel excipients. -
News Thermo Fisher Scientific expands viral vector manufacturing capabilities with Plainville facility
Thermo Fisher Scientific’s new Plainville, MA viral vector manufacturing facility has opened, expanding the company's cell and gene therapy capabilities with advancing bioproduction and analytical instrumentation technologies. -
News $475 million acquisition of Metrics Contract Services by Catalent Inc.
Catalent Inc. is set to acquire Metrics Contract Services in a USD $475 million bid to expand its high-potent capacities and oral development and manufacturing capabilities by the end of 2022
Position your company at the heart of the global Pharma industry with a CPHI Online membership
-
Your products and solutions visible to thousands of visitors within the largest Pharma marketplace
-
Generate high-quality, engaged leads for your business, all year round
-
Promote your business as the industry’s thought-leader by hosting your reports, brochures and videos within your profile
-
Your company’s profile boosted at all participating CPHI events
-
An easy-to-use platform with a detailed dashboard showing your leads and performance